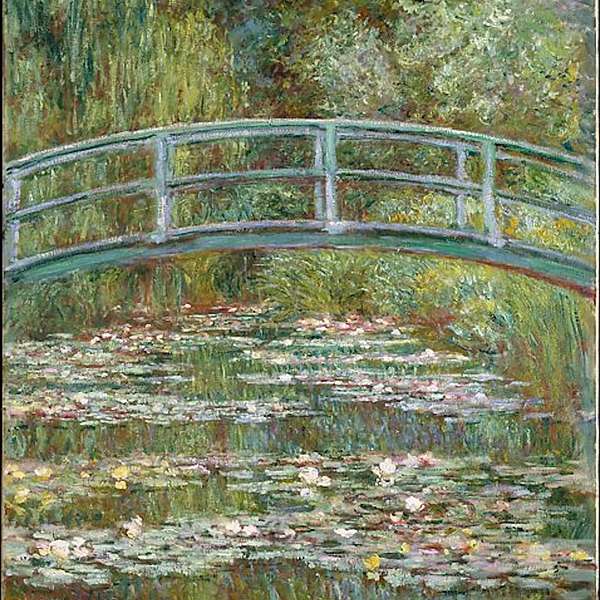
Valley Nordic
Valley Nordic
Valley Nordic S2 E30: Product Management With AI
How we develop products continues to evolve as new technologies get commercialized. Products that work with Machine Learning (ML) models require a new way of thinking to launch and improve products. The agile/lean approach does not work with ML. We discuss how product managers will have to develop products differently with ML.
Hi everyone. Welcome back. This is Chander in Palo Alto. Hello out there. This is Arna in Oslo. Anything new Arna since last week in Oslo. It's raining. It's getting darker in the evening. Otherwise, life is is the same. Are you missing California when it's dark in rainy? Yes, I do, although I don't really, I'm not really envious of orange skies and fires and covid and presidents and things. So I think all together, it's, you know, I can deal with some rain. Yeah. Okay, let's come to the topic of the day. I think last couple of episodes, we had company analysis. So this episode, we thought we covered something more operational, and that's how product development is changing with the you know, more with usage of AI or machine learning models. So, yeah, yeah, because I think more and more people are using AI or ml in everything. So this, you have to think, if you're trained in Lean, then you may have to, you know, change your mind on how to do things in the AI world. So why don't we start RNA with, what? Your idea of Lean product management or development is, our Lean Startup as some people, I mean, lean is a number of things, but in the startup context and in product development context, lean is a methodology to try to get to what customers need quickly and with least resources, and thereby getting to the position where you can start scaling. But like the core idea is that you build a product with as least as little resources as possible, which is called a minimum viable product that has like the core functionality that you think customers need based on a hypothesis of what customers need. And then you experiment with that to get customer feedback. And so you get MVP and hands on customers as quickly as possible. And then you get feedback, and preferably measurable feedback, and you iterate till customers like it and it, you know, it works. And then you have like your product to build from. That's like the up, you disappeared. Arna, I can't hear you. Is that clear? Yeah, it was silently clear. I couldn't hear you. Ah, okay. What I heard was, I think for the most part, we got what you mean by lean startup. But I would say when it applies to product development, it used to be the model was called waterfall, which is still used in many places and in products which is which are hard to iterate. It comes from the in a big system world, hardware world, I worked in semiconductor world, they still follow the waterfall model, where you plan on this is, this is the product roadmap, and these are the requirements, and you build on those requirements, and it long product development cycle, like 18 months or two years, and you mass produce when you launch. So then came the Agile Model, which is more what's used in lean startup. So lean is like kind of a startup that basically means, you know, have do a lot of stuff with limited resources, but the Agile method methodology is more around how to think about product development. So one, if you have a list of, you know, 20 requirements, you know, figure out what are the easy ones? Months, you can do in least amount of time that provide value to customer. So out of 20, maybe there's 123, that can add value to customer, and then you iterate on that. So you have these things called sprints, where you release everything one week, two weeks, three weeks to the customers, and then you keep on iterating, so it's like continuously. So that that's the general idea of Agile. Get the goal. You may have heard, you know, move fast. That's used a lot. So that's the general idea of agile, lean, basically add value to customer, not a lot, but at least, like whatever is better than competitors or that doesn't exist. And get out there, get feedback. Iterate, iterate, iterate, yeah, yeah. And I think, I think there's one added angle to this, and one of the reasons why this has become very popular is that it's kind of been proven over time that a lot of the reason why products fail is not really because you're not able to develop them. The problem is that assumptions of what customers want are wrong. And if you have that waterfall, and you sort of launch big time after a long period of time and having invested a lot of resources, you launch successfully, but nobody wants it because your assumptions were wrong. And therefore, by getting in the hands of the customers early. You get the feedback early, and you actually hit with the right things the customers are looking for, rather than developing something that nobody wants. And that's sort of like the central idea as to why it's it's working or believed to be working, yeah. So, you know, it's contextual. It doesn't always work like I cannot launch a new chip based on agile or lean. You know, you have to manufacture. It's a product cycle. You have to develop new technology. So you can't really do Lean or agile with hardware products like iPhone comes out, they're not iterating like the iteration cycle is once a year, whatever doesn't work this year they launch next year. So you they're tighter quality controls. You have to do more research on what consumers want, what might work, and lot of it is incremental improvement. So some things you know, yeah, people want you know, faster phones, better displays, better camera, of course. So like things you already know, but in hardware, when you have to actually manufacture things in mass quantity, lean or agile doesn't work. What not happening in that space is 3d printing. You can do prototypes, get feedback, and then go to mass production there. Go ahead. Yeah, no, so that's correct. I mean, you couldn't do a full iPhone or a full semiconductor chip on, you know, without going to scale. It just doesn't work for those types of products. But if we look at another product that we talked about in one of the earlier episodes with quibi, the, you know, the mobile streaming service, or whatever we should call it, right? They went, you know, the full scale approach, without testing it would seem invested billions of dollars in something that you know, didn't seem to have been any customers hands, and could probably have saved a billion dollars if it had been, Yep, that was totally they could have done the, you know, agile or MVP approach, but they didn't. They didn't even have to launch an app. They could have done a like, you know, may snap Mini or something, but I hear, did you hear they might be selling, or, you know, trying to sell assets and close it down, yeah. But I think, I think the the Lean Startup model worked great for, like, the typical startup, and, you know, in the 2000 teams, as I would call them, where, like, the main front of innovation was essentially, you know, taking new technology that was coming out, type of like cloud infrastructure, like AWS, and like web front ends or app front ends, innovating on taking new product categories and making the user experience different through these digital interfaces and through that sort of. To build something quick, digital front end, capture customer interest on that front and preferably acquire customers, and then capture some value on whatever is going on behind the scenes, right, whether that's e commerce or taxi services or whatnot, right? And And essentially, that you know where your iteration is primarily a front end software that you can build by two engineers and test it in the hands of customers. That's a very low threshold for technical risk and technical development, right? And that's why this arena that was like the hottest topic of like 2010 to 2020 sort of that type of innovation and that methodology worked really, really well because all the technical components were there available, more or less through APIs or SDKs and or open source source software, right? Yeah, it's, I think lot of the Agile or lean became possible because of internet. So pre internet and pre personal computing, it wasn't really possible to do Lean because it was mainframes and the businesses were users. They have requirements, you know, cycles, how they do upgrades, etc, and you couldn't really sell, you know, like, everything was sold to businesses. Personal computing was limited. After internet, you know, you had a website, you can just launch a new version every day. So you see, that's how it became possible. And of course, cloud accelerated the trend. And now what I see happening, smart smartphones accelerated the trend, yeah, yeah, but that's also cloud without, you know, and endpoints for clouds, yes, exactly, yeah. Now I see the trend is lot of like commercialization of the technology elements you look at Shopify, you could basically launch an E commerce business now in like 10 minutes before I remember I when I worked on my first website, long time ago, you had to get the server and, you know, get somebody to build the website. Then came, like the WordPress era, which was still messy to use. You have these plugins, and now everything's outsourced, like B to B SAS, your favorite thing. You can just Yes, but with AI coming back to AI, I think the model will have to change again. Yeah, so you've been looking into this. What? What changes do you see? When, when, sort of the trend now is AI, so I worked on products in the past few years that use machine learning models, and I see how things are different. So for example. Well, let's start with AI. AI is basically, there are two dimensions. There's like, you know, domain specific AI, and there's general AI. When we talked about GPT three, that's general AI, or, in the lingo, AGI, artificial general intelligence. So that's relatively new development with GPT three. It's so much progress, so we are not talking about that here. Here, it's more domain specific AI, and within that, machine learning based AI, which is all driven by supervised learning, where you take a large amount of data, train the model back, test and so that model can you know, predict what happens when it accounts a new variable or not, new new variable, new data set. Then there is unsupervised learning, where you train the model without data, like AlphaGo, but that's still in research phase. I don't know any products which are using pure unsupervised learning. So with that context. So this will, this is more supervised learning with data sets. So there is this, you know, concept of false positive and false negative. So for, I think many people are familiar with Alexa. Or, okay, Google or Google Assistant, where to tell you what it means. What's false positive or negative? Like, sometimes, let's say Siri, yeah, Siri wakes up. Sometimes you're talking to somebody. So that's a false positive meaning. My intent was not to talk to Siri, but every something I said to somebody else, it thought that I was talking to Siri. So that's false positive. And sometimes I say Siri, and it doesn't wake up, so that's a false negative. So that's the general context of within machine learning models, you have false positives are negative, and models are based on, you know, as I said earlier, training based on data sets. Now, what is the right amount of data set for it to be trained? And that is, you know, based, like generally, the engineers specially consider it to be a good model based on, you know, how many parameters it has, how many variables it can take in and give out the result. And there's generally a fight between product managers and ml engineers, like product people would say, oh, you know, it's not giving me the results as I expect. The model is bad. And the ML engineer would say, No, the model is good. You need to use it more so I can get more data and the model can get better with data. So it's a constant fight that happens. So then you can't really do Lean if you know the product is giving you bad results or a lot of false positives or negatives, then people won't like it and they won't try it again. So now we have to think also in terms of, is it ready? Is it at the rate of, you know, 99 whatever percent, depending on the context, how much people are willing to tolerate, and what the other options are on when you launch the product. And I don't think this, you had to think before, but now you have to think when you design the product, how is my design making the product better with more usage? So for example, in case of series, example, if, if I'm designing Siri, and I see, okay, somebody tries to say something, and I I get a false positive, then I should record that and train my model. So as a product manager, you have to think about that like these are the close variations which are happening. So I should design things that eventually it filters them out. Same thing with the negative. If I say, see it doesn't wake up, and then next time it wakes up, then I should see why I said something that it didn't wake up, and now it's waking up. So the thinking has to change about product design. So not only you have to see how you can use the ML model, you have to also think about what will make the model better with time. So that's a very different way of thinking about product development, which people haven't, well, most people haven't done in the past. In the past, you know, generally it's like, Okay, we have this idea. I have the engineers. Let's, you know, put it out there and see what happens. And then we literate This is, yeah, so let me try to see if I get it to it, you say, then try to distill, distill it, right? So in the Lean Startup sense, you would say, Okay, I think the customer wants this, which might be a feature, or something like that, right? You test it, and the customer wants that. And, you know, Aha, I know the customer wants this feature. You can write code and it prescriptively makes the system do what is specifically something that the customer wants to fill that feature that's like the, like, the general way of coding something, right? Yeah. But in in in an AI based world, the product, or the feature, the output of the system, is a function of an algorithm and the data, and it's not entirely predictive. The output is not entirely predictive. It depends on the algorithm and the data and the data changes or it might be renewed, so it's not entirely predictive. And therefore, by starting and saying specify this feature is not compatible with the way of the system working, so to speak. Yes, well, I would say it is predictive, because you see, okay, the model can give the intended result or accurate result 95% of the time, or 97 so that's predictive. So it's, you know, but it's input very It depends on how good the input is, Yeah, makes sense, right? But say you're say the customer wants a feature that recognizes cats, pictures of cats, yeah, you know, you say, you write the code and say, I would like to recognize cats, and you start putting data through it. It may or it may not recognize cats, depending on the quality of algorithm and the quality of your data. But if, if the system has done this is well trained for a long time, it will be very accurate on this task, but it's entirely unpredictable when it becomes that accurate. When what becomes accurate, the model, the system, becomes accurate in recognizing cats from dogs and other animals and not getting it wrong either way. So that is predict. So what you do is you take lot of pictures of cats in all different poses, shapes and forms, conditions, and you write an algorithm you teach the machine to recognize that this is a cat. Their edges and they're like, lot of variables going go into that the more the variable generally, the better the model, exactly. But the first time you put a picture through it, it doesn't do it. Sorry, say that again. The first time you put a picture through it, it doesn't do it because it hasn't been trained yet. Yeah, yeah, you have Yes, but it at that point, it's, I would say the first picture is probably not predictable. If it's, it's going to recognize or not. But over time, it becomes very predictable. You can pretty much predict with, let's say, that 97% of the time, 99% of time, it will recognize it. But there are certain cases like, you know you saw that Uber accident with autonomous vehicle, the model did not see, I forget what the case was, the specific case the model wasn't trained on. So that's how the accident happened with autonomous driving. But the problem will now, I think it's not like the startups are going to hire a lot of ml people and everybody's going to get develop models, get the data, train the model. I don't see things going that way. What I see is, you know, companies like open AI, or other companies. They it'll it becomes a layer in the ecosystem. Just like we have the cloud layer, we'll have aI layer, where you can get a trained model which is trained on whatever, and then you kind of prime the model for your use case, and then you release it. So that's where you have to think that for your use case, you know, how do you think about product? Are you just, you know, you can't just put it out there as fast as you can. It has to work up to certain extent that people don't, you know, give it up. It has to offer value and not annoy customers. The lot of testing and planning is required compared to the lean or agile method, where, where you don't you just put it out there. The speed is of the essence. So now speed becomes secondary. You see the primary becomes the level of accuracy on the case and how you are capturing data while usage of the product. Yeah. So I agree with you, to some extent, in that there are a lot of open source libraries doing machine learning things like so Google has been a driver for the TensorFlow framework. That's very. Popular framework from an algorithmic perspective, and there are also big data sets for training out there. So if you have a standard problem like language or recognizing animals or something like that, there is training data, and you can sort of piggyback on that development. But if you're looking to do something new. One of the big friction points in my mind is that if you want to do something new, you actually have to have a unique data set just to get something to work. And that is something that's counter to, you know, just building something and putting it out there, because getting a big data set is a lot of work, and it's hard to get proprietary data that is not a low effort exercise, to put together a proprietary data set of significant size, to train an algorithm to do something interesting, so that, I think, is one of the fundamental issues with being lean, if you go for something that's not already off the shelf, and I think your view of the world, that it becomes like light cloud component is true as a vision for the way the world is going. I just think it's much, much more immature. So it's it's quite a long way before the perfect tooling is out there and all the data sets for public training is available. Yeah, you know, startups can't spend millions of dollars getting the data and but some can you look at autonomous vehicles, they are, you know, like three, four companies spending millions and millions of dollars, dollars, and many of them giving up efforts, and it still doesn't work. Imagine if you apply lean startup methodology to, you know, autonomous vehicles doesn't work. That's absolutely correct. And I think, I think, you know, that's one of the hardest problems there is out there, to be honest, there are much simpler AI problems to solve, but I still think that the level of effort that you have to have in building something, certainly beyond the point where you just use something off the shelf, is is much more effort than, you know, building a front end and taking out there, because if, if, if you need to train something that's unique, you have to have that data, you have to process that data, And you have to have still more deep tech r&d knowledge inside the company, and that means larger team size. You have to have a more clear view on where you're going with it. And it's just much more resource intensive and skill intensive to build something that's not exactly off the shelf. So I would say that there is a big segment of startups that use AI for something that's different, but it's not at on the order of magnitude of building an autonomous vehicles, but it's also much, much more complex than building an app with a good user experience. So I think there's this middle ground of complex startups, but worthwhile startups that still fits the startup model, but not the Lean Startup model, yeah, yeah, but yeah. So of course, Tiktok is a great example. It's all working on ml models. It's, you know, great product. It's, they created their own data. They're feeding the model with the data. So I think that if you can create that loop, it works, but it requires a lot of money. It's not, you know, it's not as easy as you know, you giving a company $2 million and they create a product that people use. It requires more investment, longer time horizons, which generally VCs don't have as much appetite for. But Well, there has been a lot of investment going into AI based startups in the last three years, but, but I completely agree that they require much more resources than the traditional lean startups, and I think they require something else as well. They require some way, some access to proprietary data, or a way of capturing data to build something unique, and that is the hardest part of it, beyond the resources and skills required to do it. Yeah, or, you know, taking public data and building a better model to. You look at the, you know, in the consumer successes of AI, where you have, you have, you know, these assistants, which is big companies, Apple, Google, Amazon, you have, you know, Facebook, which is using ml models, or, you know, news feed, etc, the Tiktok, which is using ml models, but there aren't, like, small, you know, chatbots were hot, remember, like, but none of them panned out. It wasn't ready. So it it, it takes more persistence and lot of money to make these work. On the consumer side, I think B to B, their different cases, their niche applications, like Palantir, like we don't know what exactly they're doing, but apparently, some AI to make sense of data. Yeah, so, so I think that what we will see in the future is that most SaaS companies and most consumer services companies will have an element of AI is just that the end user might not be entirely knowledgeable about it, but the models that I've seen working in, including in our own portfolio, is that that the AI SaaS company, which it is in our case, applies one of two models, and they tend to be both sort of vertically oriented, right? They serve a vertical market, and they either help, you know, business customers capture data and use algorithms to process that to get an output that's valuable for the business customers. So the so the So, essentially, you piggyback on the data that the business customer gives you access to and and brings value after that for the benefit of the customer that get paid to do so. And that's like the traditional SaaS model is just that there's AI in there, and it's more complicated than just building a back end in the front end and so that. So examples are like, I've talked about this company called Acrobat before, that just images in fish farming, and helps the fish farmers make sense out of that and brings value to the fish farmers for that purpose. And we see the same model in in security applications, in ag tech, where, like capturing images and analyzing them with an AI model brings a lot of value. So that's, and you said chat bots didn't work. That is that is true to some sense, in multiple places, but we do have a very successful portfolio company. They don't call themselves a chat bot, but a virtual agent, but they serve verticals, so banks, telcos and so forth in their customer service, and they are trained on the customer service data to be much, much more accurate than the generic chatbot, right? And thereby providing much more value, simply because they serve the customer much better than waiting in line to talk to a call center and and that's language AI, but it operates on the on the data sets that the B to B customers have, so either you have to help the customer capture the data or use the data they already have to train it. Yeah, yeah. Makes sense when you're thinking of developing a product in these scenarios you described. It's different than a lean or agile methodology? Yeah, the data, you have to label the data, you make a mistake in labeling the data, you have the wrong classifier. The model doesn't work, and it's secondary. That's the whole point. Yeah, it's, a tech heavy lifting to make it work. Well, yeah, so I think, like, one big point is speed becomes secondary. Second is you now have anything you do with AI? Well, AI is to generate an ML model. Not only you're solving a customer problem. So far, you know, when you're developing products, you are focused on solving customer problems, but now everything, it's like you have two kids. You are not only solving customer problems, you're also you. Thinking about, how do I make the ML model better? So you always have to think in this, you know, two way system which is different than if you just have a, you know, software product which is not using Apple model. But that's why it's also interesting, because you have the data network effect, right? Because your product gets better just by being used. Of course, some applications or use cases, there are diminishing returns when you have data beyond a certain point. But for many, many applications, the more data you have, the better it gets. And therefore the more data. If you are early and you start getting data, your likelihood of staying ahead is huge, yeah, but that that's the point. You have to design it that way. You can design an ML based product, which where you're not capturing the data to make it better. You're basically using the existing classifiers to see it works and getting feeding more data, but enhancing and making the model more effective is not part of the product design. What I'm saying is, yeah, you have to now think of that if you want the model to be better, maybe there are additional parameters which are currently not in the model. Start getting that data with the usage of the model. So that's how the thinking has to be for you to be a good ML model product person. Absolutely, it's a good point. Yeah. All right, any other points around this that you think is worthwhile mentioning? I think it goes actually. Let me pull that up there. One day I had to think and summarize ml, what it can do. So let me pull that up. So this is the equation I wrote. It all begins with digitization of everything inside the business. So what that means is, if everything is digitized, then you have faster product development, faster customer feedback, faster market expansion, cheaper distribution, cheaper marketing, cheaper operations, better pricing and better support and better decision and all this lays the groundwork for Super supervised automation or machine learning, if the data is labeled correctly, so you see, like even before you start your ml journey, you can do all this with digitization. Yeah. So I think there's an interesting angle that I see to it, and that is that we've been through like those, 10 years of UX innovation, which is essentially, you know, putting putting services and goods at the fingertips of people on smartphone right before you had to go to the store to buy this or that, or you had to pick up your phone and call a taxi to get them to come. Right now, it's two clicks on an app, right? And that's like the UX itself. But I think, you know, the next innovation is inside all of these services, whether that's a SaaS or a consumer product, you know, AI is there and it's already in the big services is already there, sort of, and that just means the recommendation for products are better or, you know, they better understand your needs to represent better options. But I think in the in the ultimate, you know, with the ultimate access to data and and ultimate algorithms, it becomes borderline scary but very convenient. And that is, you know, Amazon or Uber will know when you're out of toilet paper, and it will just be on your doorstep, because it knew that it didn't recommend you toilet paper. It just knew that it had to be there, or Uber knows that you need a car to go there, so the car just shows up when you need there. So the actual you, the touch base UX doesn't have to be there anymore. Things just pop into your lap when you need it. That's like the ultimate automation of AI. But it's obviously a question is, Are people comfortable with that level, yeah, but that I can see so many faults positive in that model, I don't see that happening anytime soon. It's not tomorrow, yeah, I think that's far, far away. Yeah. Because just there's so many variations possible, and you get few false positives, people will just start using it, and that's why they today are recommendations and not implementations or automations. Yeah, I mean, in some cases you can do like, you need verification. Lot of the supply chain ml models there, you know, like Amazon and auto in Germany is using that like they have, they let the merge. Merchant see or manufacture the supply levels, and when it goes below certain level, they automatically do the shipment without human interfering, so that, you know, but it's a trigger that a human set up and the machine is executing on it. It's not based on that can be, that can be prescriptive logics as well. Yeah, yeah. And you can say, you know, generally, Tuesday people buy, I'm making this up, you know, toothpaste. So Tuesday is when it goes out. So there's nice, you have all this data from 10 years that shows, okay, this is when it goes down. So you just ship it. But the consumer doesn't see that. It's the B to B side. So there's no you may have few extra toothpaste stored, but it's, I wouldn't classify that well, the model is false positive, but the impact is not as bad on the consumer or the business. Yeah, and that's why I think, you know, automation will first come in. You know, toothpaste and toilet paper, because if you have an extra roll of toilet paper, is no, no danger in that. But if the Uber shows up on Tuesday instead of Wednesday, that's, that's, that's more of an issue. Yeah, even that's different toilet paper showing up at home versus showing up in Amazon warehouse. I think they're very two different contexts, like it could be false positive for the consumer, but for the business, it might be fine. So the point is highly contextual where it is applied, but for consumer side, I think it's far, far away that you basically before you think it's there. The machine knows you more than you know yourself in all different situations and scenarios. Well, I beg to differ. I think in some consumer services, it will be there very soon, and I'm thinking about very basic consumables like toilet paper and coffee and these sorts of things. And as long as they're false positives, the toilet paper come one roll early, or the coffee comes one bag early, that is absolutely fine. If the toilet roll comes one roll late, that's an issue. So they're not symmetric, whether they're false false positives or false negatives. Well, you can do that today. Subscribe on Amazon. Things come every week or month, whatever. So people are fine, yeah, but that, that's, that's, that's a time based system, not a need based system. How will the model know they use it like that's that's highly predictable, if you just have the data. But you know, that's what I mean. There's so many variations in that I travel one day and I'm not at home, but that, you know, a separate discussion, not how to develop product differently. I have another episode on that. But the point of AI product development is the speed becomes secondary, and you have to think of, you know, not annoying consumers with false positives or too many false positives or negatives. And then third is you have to always think how you can make the model better in addition to solving customer problem. So everything becomes two pronged. And I would add that it's a true AI development is a deep tech exercise, at least for now and for most use cases, it requires data, which means that the resources required are just different. Yeah, I. Of course, ml, like it's all the whole thing is based on data. Yep, okay, thanks, everybody. We'll see you next week. All right. Bye, bye, everyone. Okay, bye, bye.